Introduction to AI Language Model Comparison
The AI language model arena continues to grow more competitive with new players challenging established frontrunners. Among these contenders, Deepseek and Llama have emerged as powerful options for businesses and developers seeking advanced natural language processing capabilities. These two models represent different approaches to large language model architecture, training methodology, and real-world applications. Understanding their comparative strengths and limitations is crucial for organizations looking to implement conversational AI solutions in their operations. This analysis dives into the technical specifications, performance benchmarks, and practical applications of both Deepseek and Llama, offering insights for decision-makers exploring AI language technologies for business implementation.
Origins and Development Backgrounds
Deepseek emerged from the Beijing-based startup Deepseek AI, founded by former ByteDance researchers in 2023. The company quickly positioned itself as a serious player in the AI space with substantial funding and technical expertise. Llama (Large Language Model Meta AI), on the other hand, was developed by Meta (formerly Facebook) and first released in February 2023, with subsequent versions enhancing its capabilities. The development paths of these models reflect different corporate philosophies: Deepseek represents the rapid innovation characteristic of specialized AI startups, while Llama embodies Meta’s strategic push to establish open-source alternatives to proprietary models like GPT-4. The AI phone service industry closely monitors these developments as they directly impact the quality and capabilities of voice-based solutions.
Technical Architecture Comparison
The architectural differences between Deepseek and Llama provide fascinating insights into their respective design philosophies. Deepseek employs a modified decoder-only transformer architecture with enhanced attention mechanisms and optimized parameter efficiency. Its models range from 7B to 236B parameters, with the larger versions implementing mixture-of-experts techniques. Llama, particularly in its Llama 2 and Llama 3 iterations, utilizes a streamlined transformer architecture focused on computational efficiency, with models ranging from 7B to 70B parameters. Both employ different approaches to context window management, with Deepseek boasting longer context windows (up to 128K tokens in some versions) compared to Llama’s more modest but gradually expanding context capabilities. These architectural choices significantly impact their performance in AI voice conversations where context retention is critical.
Training Data and Knowledge Base
The knowledge foundations of these models reveal interesting contrasts. Deepseek trained on a diverse corpus including scientific papers, code repositories, and multilingual web content, with particular emphasis on technical and scientific literature. This orientation gives it notable strengths in specialized domains like programming and scientific reasoning. Llama’s training corpus, particularly for Llama 2 and Llama 3, encompasses a broad range of internet text, books, and curated datasets, with Meta claiming careful filtering for quality and safety. The prompt engineering challenges differ between these models due to their distinct knowledge bases and training methodologies. External evaluations have suggested that Deepseek demonstrates stronger performance in technical domains, while Llama shows more balanced capabilities across general knowledge tasks.
Performance Benchmarks and Evaluations
When examining quantitative performance metrics, both models show distinct patterns of strength. On standard NLP benchmarks like MMLU (Massive Multitask Language Understanding), Deepseek-Coder 33B achieved impressive scores of 67.3%, while Llama 3 70B reached comparable performance at 68.9%. For reasoning tasks like GSM8K (grade school math problems), Deepseek exhibits particularly strong performance, with its larger models surpassing 80% accuracy. Llama demonstrates competitive performance in common sense reasoning benchmarks and shows strong results in human preference evaluations. For businesses developing AI call assistants, these benchmarks provide crucial information about which model might better handle complex customer interactions requiring robust reasoning capabilities.
Multilingual Capabilities Assessment
The global applicability of these models depends significantly on their multilingual proficiency. Deepseek demonstrates notable strengths in Asian languages, particularly Chinese, Japanese, and Korean, while maintaining solid performance across European languages. This reflects its training on diverse multilingual corpora. Llama, especially in its latest iterations, shows improved multilingual capabilities compared to earlier versions, though with somewhat uneven performance across non-English languages. Independent evaluations suggest that Deepseek maintains more consistent quality across languages, while Llama shows stronger English-language performance with gradually improving capabilities in other languages. For international AI call center operations, these multilingual differences can significantly impact customer experience and operational effectiveness.
Code Generation and Technical Applications
In the specialized domain of code generation, significant differences emerge. Deepseek-Coder, specifically designed for programming tasks, demonstrates exceptional code generation capabilities across multiple languages including Python, JavaScript, Java, and C++. Independent evaluations have shown it outperforming many competitors on benchmarks like HumanEval and MBPP. Llama’s code generation capabilities, while improved in recent versions, generally lag behind specialized models like Deepseek-Coder in both accuracy and complexity of generated solutions. For technical applications requiring code integration with conversational AI systems, Deepseek’s superior code generation capabilities may offer significant advantages for development teams.
Instruction Following and Response Quality
The ability to follow nuanced instructions and generate helpful, accurate responses varies between these models. Deepseek models show strong instruction-following capabilities, with particularly good performance on complex, multi-step instructions. Their responses tend to be comprehensive and technically precise, though sometimes lacking conciseness. Llama, especially Llama 3, demonstrates impressive improvements in instruction following compared to previous versions, with notable strengths in generating balanced, aligned responses that reflect subtle nuances in prompts. For applications like AI sales representatives, these differences in instruction following can significantly impact conversion rates and customer satisfaction.
Licensing and Commercial Usage Considerations
The legal frameworks governing these models present important considerations for business implementation. Deepseek offers dual licensing options: its base models are available under the More Permissive License (MPL), allowing for commercial use with certain restrictions, while also offering commercial licenses with more flexible terms for enterprise applications. Llama 2 introduced Meta’s community license, allowing commercial use with certain limitations, particularly for systems with over 700 million users. Llama 3 continues this approach with some refinements. For businesses developing white-label AI voice agents, these licensing differences directly impact the commercial viability and scalability of their products.
Deployment Requirements and Resource Efficiency
The computational demands of these models present practical implementation challenges. Deepseek models, particularly the larger versions, require substantial computational resources, though the company has worked to optimize performance for deployment scenarios. Their mixture-of-experts architecture in larger models aims to improve efficiency without sacrificing performance. Llama models are specifically designed with deployment efficiency in mind, with Meta emphasizing optimal performance on consumer hardware. The smaller parameter count of Llama models (even at 70B vs. Deepseek’s larger models) results in lower memory requirements and faster inference speeds on comparable hardware. These efficiency differences directly impact operational costs for AI call center solutions where response time and throughput are critical metrics.
Fine-tuning Capabilities and Customization
Adaptability to specific use cases through fine-tuning represents a key consideration for specialized applications. Deepseek provides comprehensive support for fine-tuning across various tasks, with particularly strong results for domain-specific applications in technical fields. Their models maintain stability during fine-tuning with less tendency toward catastrophic forgetting. Llama offers robust fine-tuning capabilities with extensive documentation and community support, benefiting from Meta’s research into efficient adaptation techniques like LoRA and QLoRA. For businesses creating specialized AI voice assistants, these fine-tuning differences directly impact the development timeline and performance ceiling of their customized solutions.
Safety Features and Ethical Considerations
As deployment of these models expands, their safety mechanisms become increasingly important. Deepseek implements content filtering and bias mitigation strategies, though with less public documentation about specific alignment techniques compared to some competitors. Llama, particularly Llama 2 and beyond, places significant emphasis on safety through a process Meta calls "constitutional AI" and RLHF (Reinforcement Learning from Human Feedback). Meta has published detailed information about their safety testing methodology. Independent evaluations suggest that Llama may have more consistent safeguards against harmful outputs, while Deepseek occasionally demonstrates more variable responses to potentially problematic prompts. For customer service applications, these safety differences directly impact brand protection and regulatory compliance.
Integration Capabilities with Voice Technologies
The integration potential with voice technologies represents a crucial consideration for telephone-based AI applications. Deepseek demonstrates strong natural language understanding capabilities that translate well to voice applications, though with less specific optimization for speech-to-text and text-to-speech integration compared to some alternatives. Llama has benefited from Meta’s broader ecosystem of voice technologies, with emerging work on multimodal capabilities that bridge text and speech. Third-party integrations have shown both models can work effectively with platforms like ElevenLabs and Play.ht for high-quality voice synthesis. For businesses developing AI phone agents, these integration capabilities directly impact the naturalness and effectiveness of customer interactions.
Community Support and Development Ecosystem
The surrounding ecosystem significantly impacts implementation support and future development. Deepseek has a growing but smaller developer community compared to Llama, with most resources directly provided by Deepseek AI. Their documentation is comprehensive but primarily focused on technical aspects rather than application development. Llama benefits from Meta’s extensive resources and a large, active open-source community, with extensive documentation, tutorials, and third-party integrations. The vibrant Llama ecosystem includes numerous adaptation projects, fine-tuning examples, and deployment solutions. For businesses without extensive AI development teams, these ecosystem differences can significantly impact the feasibility of implementing solutions like AI appointment schedulers or call answering services.
Real-world Application Performance in Call Centers
The practical performance in call center environments reveals important operational differences. Deepseek demonstrates exceptional technical knowledge handling and complex query resolution, making it particularly effective for technical support scenarios requiring deep domain knowledge. Its longer context window enables it to maintain coherence throughout extended customer interactions. Llama shows strengths in maintaining conversational coherence and natural dialogue flow, with good performance in multi-turn conversations that characterize customer service interactions. Its alignment tuning results in consistently professional tone and approach. Organizations implementing AI call center solutions report that the choice between these models often depends on the specific nature of their customer interactions, with technical support favoring Deepseek and general customer service sometimes benefiting from Llama’s conversational fluidity.
Integration with Business Systems and CRMs
The enterprise integration capabilities of these models present different implementation challenges. Deepseek offers API access with flexible integration options, though with less comprehensive official connectors for common business systems compared to some alternatives. Third-party developers have created integration solutions for popular platforms, but implementation often requires more custom development. Llama benefits from Meta’s broader commercial ecosystem and extensive third-party support, with numerous pre-built integrations for CRM systems, customer service platforms, and telephony services. For businesses looking to implement AI phone consultants that connect seamlessly with existing systems, these integration differences directly impact implementation timelines and ongoing maintenance requirements.
Cost Analysis for Commercial Implementation
The financial implications of choosing between these models deserves careful consideration. Deepseek’s hosting and inference costs typically run higher than Llama’s due to larger parameter counts in its most capable models, though this difference narrows with their smaller model variants. Commercial licensing for Deepseek can represent significant investment for enterprise deployments, while self-hosting options provide more cost control. Llama’s permissive licensing and focus on efficiency typically results in lower total cost of ownership, particularly for self-hosted deployments. Cloud API options for both models through providers like OpenRouter offer usage-based pricing that can be more economical for lower-volume implementations. For businesses evaluating AI cold calling solutions, these cost differences directly impact ROI calculations and deployment strategies.
Future Development Roadmaps and Innovation Potential
The future trajectories of these models offer insights into their long-term viability. Deepseek has announced ambitious development plans focusing on larger, more capable models with enhanced multimodal capabilities and specialized vertical applications. Their research publications suggest continued focus on technical domains and reasoning capabilities. Meta’s roadmap for Llama includes continued improvements in efficiency, multilingual capabilities, and multimodal extensions, with particular emphasis on responsible deployment and broader ecosystem integration. The company’s scale suggests long-term commitment to the platform. For businesses building AI calling agencies or long-term voice automation strategies, these roadmap differences directly impact platform sustainability and future capability expansion.
Model Comparison for Specific Voice Agent Use Cases
The suitability of these models varies significantly across specific voice agent applications. For appointment setting tasks, Llama often demonstrates superior performance in understanding natural scheduling requests and managing calendar operations, making it well-suited for AI appointment booking bots. For technical support scenarios, Deepseek’s exceptional technical knowledge and reasoning capabilities provide advantages in troubleshooting complex problems. In sales contexts, Llama’s balanced conversational abilities and alignment tuning often result in more persuasive interactions for AI sales calls, while Deepseek’s technical precision can be advantageous for complex product explanations. Healthcare applications typically favor models with strong reasoning and knowledge capabilities like Deepseek for AI calling bots in health clinics, though with careful attention to compliance and safety considerations.
Conclusions and Strategic Implementation Guidance
After thorough analysis, several strategic recommendations emerge for organizations evaluating these models. For technically complex domains requiring deep knowledge and reasoning, Deepseek often provides superior performance, particularly with its larger model variants. For general customer service, sales, and conversational applications where natural dialogue flow and balanced responses are paramount, Llama typically offers advantages, especially considering its efficiency and ecosystem support. Many organizations find hybrid approaches optimal, leveraging each model’s strengths for different aspects of their operations. The integration capabilities, licensing considerations, and deployment requirements should be carefully evaluated against specific business needs. As these models continue to evolve, organizations should establish robust evaluation frameworks to periodically reassess their technological choices in this rapidly advancing field.
Taking Your AI Voice Solutions to the Next Level
If you’re looking to harness the power of advanced language models like Deepseek and Llama for your business communications, Callin.io offers a streamlined solution. This platform enables you to implement AI-powered phone agents that can handle incoming and outgoing calls autonomously. Whether you need capabilities better suited to Deepseek’s technical precision or Llama’s conversational fluidity, Callin.io’s flexible infrastructure supports integration with cutting-edge language models to create natural, effective voice interactions.
The free account on Callin.io provides an intuitive interface for configuring your AI agent, with test calls included and access to the task dashboard for monitoring interactions. For those seeking advanced features like Google Calendar integrations and built-in CRM functionality, subscription plans start at just 30USD per month. As language models continue to advance, Callin.io ensures your business stays at the forefront of AI communication technology. Discover more about Callin.io and start transforming your business communications today.
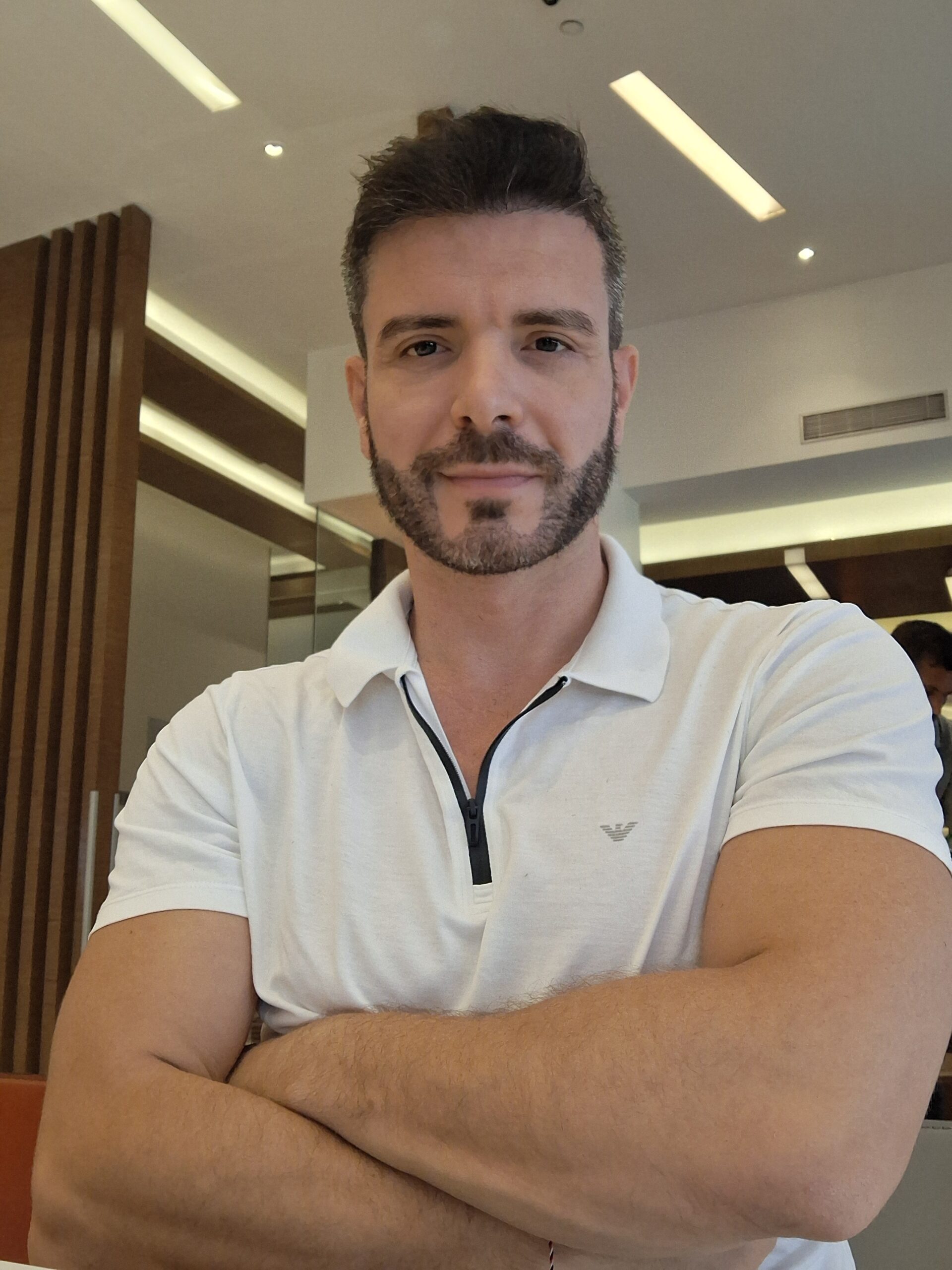
Helping businesses grow faster with AI. π At Callin.io, we make it easy for companies close more deals, engage customers more effectively, and scale their growth with smart AI voice assistants. Ready to transform your business with AI? π Β Letβs talk!
Vincenzo Piccolo
Chief Executive Officer and Co Founder